Uncovering JAPA
A Framework to Strengthen Transportation Planning
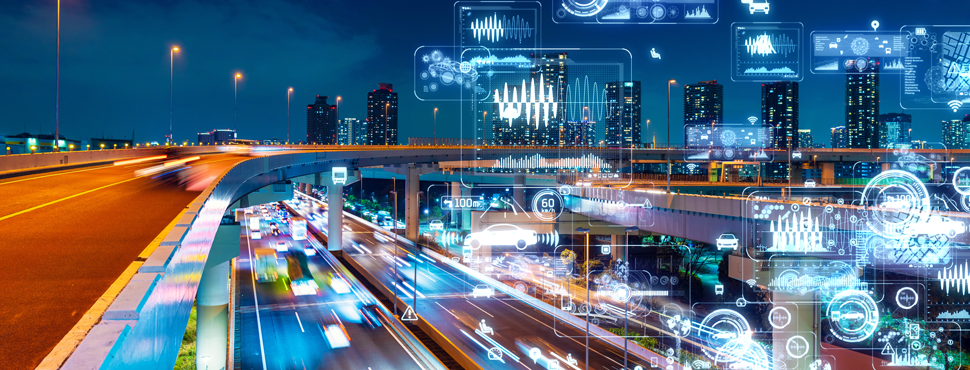
How can planners improve their long-range planning capabilities as rapidly changing social, technological, and economic factors make it more difficult to use simulation models to reliably predict the future?
This is the question that Robert Lempert and six coauthors help to answer in "Meeting Climate, Mobility, and Equity Goals in Transportation Planning Under Wide-Ranging Scenarios" in the Journal of the American Planning Association (Vol. 86, No. 3).
RDM Strengthens Planning Through Scenario Analysis
The authors examine a framework known as robust decision making (RDM), which is "a set of quantitative scenario-based decision analytic methods and tools for informing decisions under conditions of deep uncertainty."
The RDM framework differs from predictive scenario planning most commonly used in transportation planning now, which uses best-estimate forecasts of relevant explanatory factors and sensitivity testing to assess changes to only one or two assumptions at a time.
While also using scenarios, RDM is differentiated as an exploratory (rather than a predictive) framework that runs systems simulations hundreds of thousands of times to stress test proposed strategies, and thus helps planners make their plans stronger and more flexible.
Though used previously in areas like water systems, the RDM framework has not been applied extensively in transportation.
To exemplify its strengths, the authors apply the RDM framework to the current regional transportation and land use plan of the Sacramento Area Council of Government, the Metropolitan Transportation Plan / Sustainable Communities Strategy (MTP/STS). First, the authors identify relevant policy levers, major uncertainties and their relationships to each lever, and measures of success with associated goal values. They then run simulations linking these levers and uncertainties and apply a "scenario discovery" classification algorithm to "parse the database into a small number of clusters differentiated by a small number of key driving forces," based on whether each model achieves its goal values.
This figure from the article shows the percentage of uncertain futures in which the plan will achieve each goal:
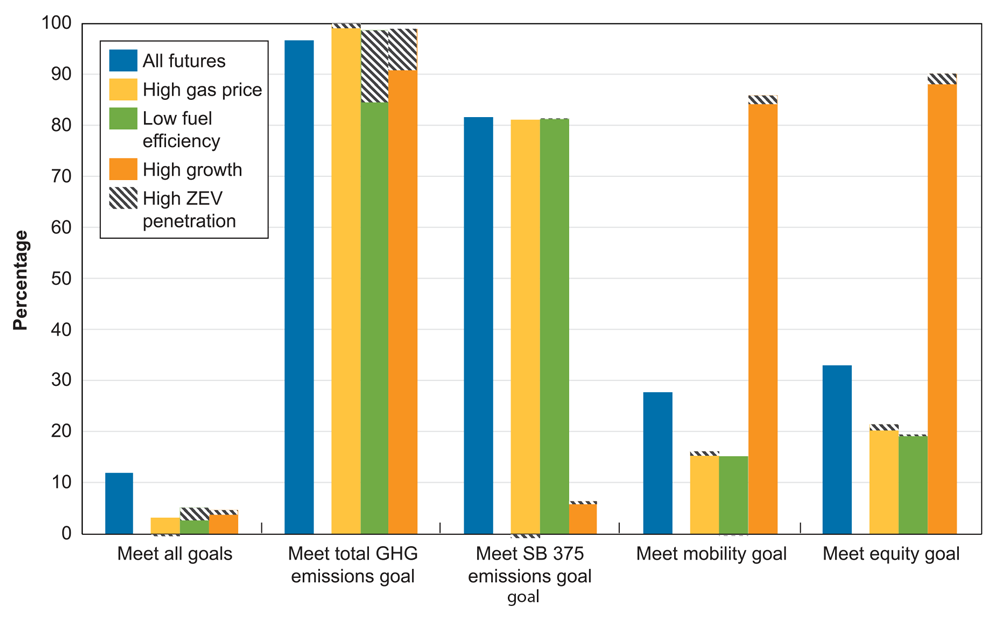
Figure 3: Fraction of futures in which 2016 MTP/SCS meets goals in alternative scenarios.
Framework Identifies Plan Success Factors
This method allows the authors to find that the plan will usually meet its goals in the future with low gas prices, high fuel efficiency, medium economic growth, and high sensitivity amongst residents to the cost of driving. It also helps them find more specific relationships between policy levers and uncertainties; for instance, the plan is unlikely to meet its mobility goals in a high gas price scenario or its total GHG emission reduction goals in a low fuel efficiency scenario.
It is these particular relationships between levers and uncertainties that show the framework's strength, as planners can then focus more resources on a particular area of the plan or be ready to adjust methods with shifts in real-world factors.
Ultimately, the authors do not advocate for solely using RDM in favor of predictive scenario planning; rather, they suggest introducing RDM parallel to existing predictive scenario methods to stress test scenarios and to help agencies explore how they can incorporate new methods.
Though discussed here in the context of transportation, this method can be used by any systems-focused planners as they seek to explore the likelihood of thousands of future scenarios and how to plan in the face of deep uncertainty.
Top image: Getty Images photo.